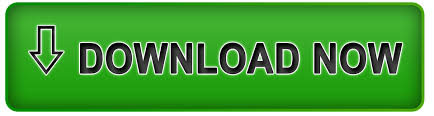
Master’s thesis, University of Portoĭawson, R. Online network analysis of stock markets. One trillion edges: Graph processing at facebook-scale. SIAM Journal on Matrix Analysis and Applications, 33(4), 1272–1299.Ĭhing, A., Edunov, S., Kabiljo, M., Logothetis, D., & Muthukrishnan, S. On tensors, sparsity, and nonnegative factorizations. Using congestion zones for solving the time dependent vehicle routing problem. Understanding search engines: Mahematical modeling and text retrieval. In: Advances in Social Networks Analysis and Mining (ASONAM), 2013 IEEE/ACM International Conference on, pp. Network similarity via multiple social theories. īerlingerio, M., Koutra, D., Eliassi-Rad, T., & Faloutsos, C., (2013). SIAM Journal on Scientific Computing, 30(1), 205–231.īader, B.W., & Kolda, T.G., et al. Efficient MATLAB computations with sparse and factored tensors. Data mining and knowledge discovery, 29(3), 626–688.īader, B.
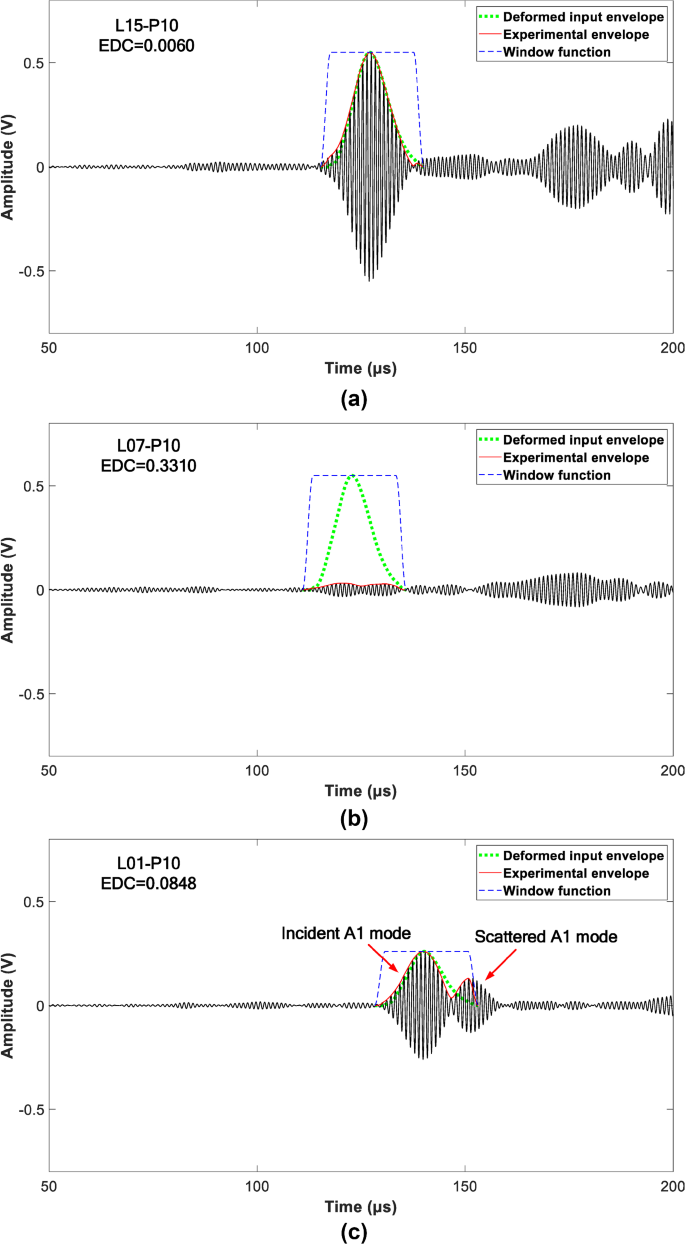
Graph based anomaly detection and description: A survey.
77 million paintings windowed mode series#
Event detection in time series of mobile communication graphs. According to our experimental evaluation, WINTENDED is able to spot global densification events at least as accurately as its competitors, while also being able to find local densification events, on the contrary to its competitors.Īkoglu, L., & Faloutsos, C., (2010). Our method combines a sliding window decomposition with statistical tools to capture the local dynamics of the network and automatically find the irregular behaviours. In order to address this issue, we propose WINdowed TENsor decomposition for Densification Event Detection (WINTENDED) for the detection and characterization of both global and local densification events. These events can occur at a global level, involving the majority of the nodes in the network, or at a local level involving only a subset of nodes.While global densification events affect the overall structure of the network, the same does not hold in local densification events, which may remain undetectable by the existing detection methods. Densification events in time-evolving networks refer to instants in which the network density, that is, the number of edges, is substantially larger than in the remaining.
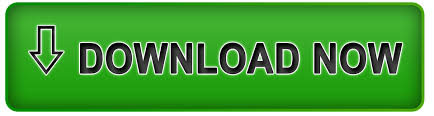